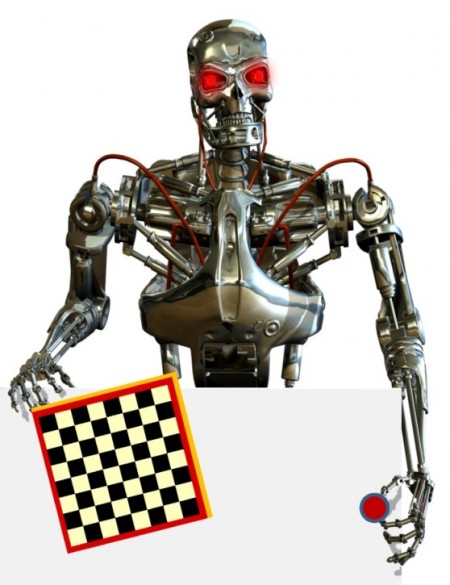
Podcast: Play in new window | Download | Embed
Episode Summary:
In this episode, we explain how to build a machine that learns to play checkers. The solution to this problem involves several key ideas which are fundamental to building systems which are artificially intelligent.
Show Notes:
Hello everyone! Welcome to the second podcast in the podcast series Learning Machines 101. In this series of podcasts my goal is to discuss important concepts of artificial intelligence and machine learning in hopefully an entertaining and educational manner.
In the real-world, every day we make many decisions at home, at work, or at play. Some of these decisions may have important consequences for our lives yet we make such decisions without actually understanding their implications. And, once we have experienced those consequences, that may influence how we make decisions in the future. Moreover, we often make decisions which we have never previously encountered before. These aspects of human behavior which include the ability to make decisions in new situations and the ability to learn from experience are important characteristics of human intelligence.
One important goal of artificial intelligence is the development of learning machines which can mimic such human behaviors. For example, consider a learning machine which learns from experience how to land a helicopter. The learning machine will be exposed to a variety of novel situations and must continually make adjustments to the controls of the helicopter, yet the learning machine’s success at landing the helicopter can not be evaluated until the helicopter has landed. At that point, the learning machine is provided feedback regarding the quality of its landing and somehow that information must be learned so that the learning machine will be more effective at landing helicopters in the future.
The methods used in artificial intelligence for training such learning machines have been around since the early days of computing. The first digital computer whose name was ENIAC
(Electronic Numerical Integrator And Computer) was developed in the early 1950s. In the late 1950’s, the scientist and engineer Arthur Samuel was interested in exploring fundamental issues of artificial intelligence. Specifically, the problems of learning from experience and making decisions in new situations. Samuel decided to explore these ideas in a more simplified setting by developing a computer program that could play checkers.
Checkers is a board game which is much simpler in its complexity than the board games of Chess and GO yet more complex than the board game tic-tac-toe. Each player moves a playing piece on the board according to specific rules and tries to “capture” or “block” the opponents playing pieces. When one player can not make a move, then that player loses the game and the other player wins the game.
Real-world problems can be viewed as more complex versions of board games such as checkers. In the checker board world, one has a particular configuration of playing pieces on the checkerboard. Such a configuration can be interpreted as a simplified model of a more complex real-world situation typically encountered by a biological (or artificial) intelligence. So, like the checker board world, the real-world can be characterized at a particular moment in time by the concept of a situation. In the checkerboard world, the situation is quite simply specified by placing some pieces in acceptable locations on a checkerboard. In the real-world, a situation is considerably more complicated yet nevertheless the essential idea of a situation is the same for both the checkerboard world and the real-world.
Another point of similarity between the checkerboard world and the real world is the concept of a “move” in a checkerboard game. Making a “move” in a checkerboard game corresponds to making a “decision” in the real-world. In the real-world we make thousands of decisions each day which could have important consequences for us in the future and in many cases we don’t understand the implications of those decisions until much later. Similarly, in the checkerboard world, decisions are made regarding which checker piece to move or which of the human’s checker pieces should be captured and the consequences of those decisions may not be understood until much later in the game. However, unlike the real-world, the outcome of a checkerboard game is easily interpretable as either a “win”, a “loss”, or a “draw”. In the real-world, it may be much more difficult to determine whether a particular situation is a “winning” or “losing” situation.
In summary, there is a close correspondence between the problem of learning to play checkers and the problem of learning to make intelligent decisions in the real-world when the outcomes of those decisions are not available until the distant future.
One method for building a machine that can play checkers is to use a Look Ahead Strategy. To illustrate this concept, suppose the learning machine and the opponent will always have 5 possible moves at each turn. In practice, the number of possible legal moves one can make will vary dramatically from turn to turn. The learning machine realizes that it can make 5 possible moves and for each of those 5 possible moves, the opponent can make 5 possible moves. That leads to 25 possible checkerboard situations. To make things even simpler, suppose that 2 of those 25 possible checkerboard situations is a “win” for the learning machine. Then, it seems that it should be straightforward for the machine to “backtrack” from that winning checkerboard situation and figure out what sequence of moves it should make to obtain the goal of winning the checkerboard game. However, not so fast, … there is something else we need to worry about.
If we look closely at the sequence of moves leading to the first winning checkerboard situation we see that we were assuming that the opponent would make a move that would “help the learning machine win” the checkerboard game. On the other hand, for the second winning checkerboard situation we see that we are assuming that the opponent would make a move that “avoids helping the learning machine win”. In practice, the opponent will always try to win so the learning machine doesn’t want to consider a sequence of moves which is based upon the assumption that the opponent is going to help the learning machine win. In fact, the learning machine might assume that the opponent is also a learning machine and is also doing a Look Ahead Strategy. Under this assumption the machine deduces that whenever the opponent makes a move, the opponent will try to move a checker piece so that ultimately the machine will lose and the opponent will win. In this manner, the machine can actually predict how his opponent would move in response to his move by making the assumption that the opponent is just as smart as he is! This type of analysis lets the machine figure out what move would be a good move if he “looks ahead” 2 moves in order to see the 25 resulting checkerboard positions.
This seems like a pretty good strategy but there is still another problem. The length of a checkerboard game is about 50 moves. If we assume that both the machine and the opponent always have about 5 possible moves, this means that after the machine moves and then the opponent moves there will be 25 possible (5 times 5) checkerboard situations. If we go another turn corresponding to the case where the machine moves, the opponent moves, the machine moves, and then the opponent moves this results in 625 possible (5 times 5 times 5 Times 5) possible resulting checkerboard situations. After 50 moves, there will be virtually an infinite number of resulting checkerboard situations. Even a computer which could analyze 1,000,000 checkerboard situations in 1 second would take centuries to consider all possible sequences of checkerboard moves!
In order to improve the performance of the look-ahead strategy, we now introduce the concept of an Evaluation Rule. This is a powerful idea which is the basis of the majority of many popular approaches to building artificially intelligent machines. The basic idea is that you give the Evaluation Rule a Checkerboard situation, and the Evaluation Rule gives you back a RATING which is a number. If the RATING is small, this means that the Checkerboard Situation is a winning situation. If the RATING is large, this means that the Checkerboard Situation is a losing situation.
In order to define the concept of an Evaluation Rule we need to introduce still another idea which is also fundamental to the field of artificial intelligence. This is the concept of a feature. If you have a lot of checker pieces and your opponent has a few checker pieces then you are doing well so we would say you are in a good situation and the evaluation rule should assign a rating such as 2 to the checkboard situation. On the other hand if you have only a few checker pieces and your opponent has a lot of checker pieces then the evaluation rule would assign a rating such as 100 to the checkboard situation. We would call the “number of checker pieces that you have on the board” an example of a feature.
But the number of checker pieces on the checkerboard is not the only important feature. For example, if you have more Kings than your opponent, then we might say you are in a good situation even though you have fewer pieces. This is an example of another type of feature.
We would like to somehow combine these two different types of features: (1) the number of checker pieces owned by each player, and (2) the number of Kings owned by each player. Its unclear which of these features is more important than the other so for now let’s assume they are equally important for figuring out whether or not a checkerboard situation is a good situation for you or a poor situation. The Evaluation Rule combines this information together to generate a rating for a checkerboard situation.
An important point. It is sometimes challenging to come up with good features. For example, suppose that we counted the total number of checker pieces in the left-hand corner of the checkerboard and did not distinguish the pieces that belong to the machine from the pieces that belong to the opponent. This would be considered to be a less effective (possibly even a bad) feature because it wouldn’t be helpful in coming up with a good rating. Indeed, there are many features which are essentially irrelevant to evaluating the board situation such as the quality of the checkerboard’s construction. In many problems in machine learning and artificial intelligence we will see that picking the right features is often one of the most important ingredients in developing smart machines.
So, to summarize, we have introduced the idea of a look-ahead strategy but this strategy is limited because we can’t consider all possible sequences of moves into the future. We then talked about the idea of an evaluation rule which provides a RATING indicating whether a particular checkerboard position is GOOD or BAD. The board evaluation rule is based upon the concept of features.
These ideas may now be combined to create a more limited yet more computationally tractable look-ahead strategy. This strategy is based upon three ideas:
(1) we are always going to look-ahead 3 or 4 moves
(2) After 3 or 4 moves if the board evaluation rule tells us that the checkerboard situation
is very dismal for us on a particular move sequence, then we won’t “look-ahead” further
on that move sequence.
(3) We will stop no matter what after looking-ahead for 11 moves even if all 11 checkerboard
situations look pretty good to us using the board evaluation rule.
This type of strategy is very computationally tractable, and instead of taking the learning machine centuries to make a decision…the learning machine can make a decision within a reasonable amount of time such as a few seconds.
So we now have a method that the learning machine can use to make decisions about current situations whose outcomes are not apparent until the distant future!
However we still have the final piece of the puzzle which is how do we combine the board features in such a way to come up with a good RATING? This is where the “learning by experience” aspect of the checkerboard playing learning machine comes into play. The idea is very simple, suppose the machine is looking ahead on a particular move sequence 6 moves into the future and the RATING of the checkerboard situation 6 moves into the future is very good (e.g., a rating=4). This means that we would like the Board Evaluation Rule to tell us that the CURRENT checkerboard situation should also have a rating of 4 since if we make the best possible moves and the opponent makes the best possible moves then we would end up 6 moves into the future with a checkerboard situation rating equal to 4. However, if the CURRENT checkerboard situation has a rating of a 3 we need to adjust the calculations associated with the Evaluation Rule so that when the machine encounters the CURRENT checkerboard situation in the future the machine is more likely to assign it a rating of a 4 rather than a 3.
This type of learning is called temporal reinforcement learning because the information regarding the performance of the learning machine is not provided immediately but only provided in the future.
The principles underlying this checkerboard learning machine problem are fundamentally important ideas that are central to many modern approaches to artificial intelligence in the 21st century.
To summarize, those key ideas are:
(1) the concept of considering every possible move sequence into the future
but using an Evaluation Rule to eliminate exploring move sequences which
are not likely going to lead to good solutions. This is called Best-First Search
in the Artificial Intelligence Literature.
(2) the use of an Evaluation Rule for not only deciding which move sequences are worth exploring but also to decide which checkerboard situations are GOOD (i.e., winning situations) and which are BAD (i.e., losing situations).
(3) the importance of Features for representing the essential conceptual components of a
problem in artificial intelligence.
(4) a Learning Rule which compares the difference between the predictions of the Evaluation Rule and actual observed outcomes and then uses this discrepancy to make small adjustments to the Evaluation Rule mechanism.
It’s also important to note that the methods in which this learning machine uses to play checkers are not entirely human-like. So, for example, a computer can look ahead 11 moves into the future
and thus consider millions of possible board situations but this is something that humans can not easily do without additional tools. On the other hand, the learning procedure used by the machine can be shown to be consistent with modern theories of biological and behavioral learning in humans. The show notes at: www.learningmachines101.com provide some helpful references to this literature.
Your laptop computer is infinitely more powerful than the computers they used in the 1950s and yet….the key principles of Artificial Intelligence which were implemented in this checker playing program using 1950s computer technology are still widely used today.
Moreover, aspects of this technology are now providing neuroscientists with guidance regarding what types of neural mechanisms might be the basis for biological human learning and biological human intelligence. They are additionally providing mathematical psychologists with guidance in the development of mathematical theories of human behavior.
And finally, note that the Checker playing learning machine is essentially learning a collection of rules such as: In this situation, I should move my checker piece in this particular manner. In the next two podcasts, we will look more closely at the idea of knowledge representation using logical rules.
Further Reading:
1. This is a good reference for example work in the area of temporal reinforcement learning.http://www.scholarpedia.org/article/Reinforcement_learning
2. This is a good reference for “best-first search”: http://en.wikipedia.org/wiki/Best-first_search
3. An original description of Samuel’s Checker playing program written by Samuels in 1959 may be found in:Arthur, Samuel (1959). “Some Studies in Machine Learning Using the Game of Checkers” (PDF). IBM Journal 3 (3): 210–229.
This was reprinted recently in the IBM Journal of Research and Development (Vol. 44, Issue 1.2) in the January, 2000. There’s also a free copy you might be able to find on the web!!! Also check it out for free from your local university library (not public library or community college library).
4. The book The Quest for Artificial Intelligence by Nils Nilsson (Cambridge University Press) has a nice discussion of Samuel’s Checker playing program on pages 90-93. Also on pages 415-420 there is a nice discussion of temporal reinforcement learning. On pages 81-85 there is a discussion of the “best-first search” strategy although it is not explicitly referenced as such.
5. The book “Artificial Intelligence: Foundations of Computational Agents” by Poole and Mackworth (2010) (Cambridge University Press) is freely available (can be downloaded for free at: http://artint.info/html/ArtInt.html) This book has discussions of “heuristic search” and “features” as well as other relevant topics.
Here are some recent articles that discuss how research in artificial intelligence is influencing current theories of how information is processed and learned in the human brain (and vice-versa!). These articles may be a little technical but I provide them to help provide a snapshot of the latest research in this area!!!!
6. Wilson, Takashashi, Schoenbaum, and Niv (2014). Orbitofrontal Cortex as a Cognitive Map of Task
Space. Neuron, 81, 267-279.http://www.cell.com/article/S0896-6273(13)01039-8/abstract
7. Niv, Y. (2009). Reinforcement learning in the brain. Journal of Mathematical Psychology, 53, 139-154.
8. Sutton, R. S., and Barton, A. (1988). Reinforcement learning: An introduction. MIT Press.
Copyright Notice:
Copyright © 2014 by Richard M. Golden. All rights reserved.
I just started listening and how you break everything down is incredible. Thank you so much. Also the show notes with additional resources is golden (no name pun intended).
Thanks, I appreciate your support!!! 🙂